Research Article
Volume 1 Issue 1 - 2017
Autonomic Function Assessment in Post Traumatic Brain Injury patients with Yoga Practicing Using Kernel Method and Entrainment Techniques
Associate Professor, MET Department, Tennessee Tech University, Cookeville, USA
*Corresponding Author: Ahmed K Kamal, Associate Professor, MET Department, Tennessee Tech University, Cookeville, USA. Email id: AKamal@tntech.edu.
Received: June 24, 2017; Published: July 10, 2017
Abstract
The experimental procedure of lowering and raising a leg while the subject in supine position is considered to stimulate and entrain the autonomic nervous system of fifteen patients with Post Traumatic Brain Injury (PTBI) practicing Yoga and fifteen age and sex matched control PTBI non Yoga practicing patients. The assessment of autonomic function for each group is achieved using an algorithm based on Volterra kernel estimation. By applying this algorithm and considering the process of lowering and raising a leg as stimulus input and the Heart Rate Variability signal (HRV) as output for system identification, a mathematical model is expressed as integral equations. The integral equations are considered and fixed for PTBI Patients without Yoga practicing and TBI Yoga practicing patients so that the identification method reduced to the determination of the values within the integral called kernels, resulting in an integral equations whose input-output behavior is nearly identical to that of the system in both Control TBI without yoga practicing patients and PTBI Yoga practicing patients. The model for each group contains the linear part (first order kernel) and quadratic part (second order kernel). A difference equation model was employed to represent the system for both control PTBI patients without Yoga practicing and PTBI Yoga practicing patients. The results show significant difference in first order kernel (impulse response) and second order kernel (mesh diagram) for each group. Using first order kernel and second order kernel, it is possible to assess autonomic function qualitatively and quantitatively in both groups.
Keywords: Autonomic function; Volterra kernel; Entrainment; System identification
Introduction
Impaired autonomic function has been associated with an increased risk of mortality in Post Trauma Brain Injury (PTBI) patients [1-8]. Autonomic dysfunction involving both sympathetic and parasympathetic systems has also been demonstrated in PTBI using cardiovascular reflex tests based on heart rate to various stimuli [1-6]. However, the clinical significance and pathophysiology of these findings in PTBI are poorly understood. Conventional time and frequency domain analysis techniques based on the linear fluctuation of heart rate insufficient in outline the changes in heart rate dynamics [7-8], therefore, new methods based on nonlinear dynamics have been introduced to quantify complex heart rate dynamics and complement conventional measures of its variability.
One aim of this study is to propose another approach using Volterra kernel for system identification of nonlinear relationship between input stimulus (lowering and raising leg) and the output (HRV signals) to assess the autonomic function of control PTBI patients without Yoga practicing and Yoga practicing PTBI patients. Also, in this study, we propose simple experimental procedure to stimulate the autonomic nervous system by subjecting both groups to stimulus based on lowering and raising a leg as shown in figure 1 [15].
This study may help in screening the autonomic neuropathy noninvasively specially in PTBI patients in qualitative and quantitative way
Patients and Methods
Patients
Fifteen consecutive patients PTBI Yoga practicing Patients fulfilling disease society Brain Bank [9] clinical criteria were included in the study from patients that were referred to the department of Neurology in Johns Hopkins University. The patients were independent in their daily activities the control group consisted of 15 healthy age matched subjects selected from PTBI patients who did not practicing Yoga. They all underwent complete physical examination and has no disease or medication affecting autonomic nervous system (ANS) in their history. All subjects agreed to participate in the research prior to their inclusion in the study and the consent of ethical committee was obtained and approved the study protocol.
Fifteen consecutive patients PTBI Yoga practicing Patients fulfilling disease society Brain Bank [9] clinical criteria were included in the study from patients that were referred to the department of Neurology in Johns Hopkins University. The patients were independent in their daily activities the control group consisted of 15 healthy age matched subjects selected from PTBI patients who did not practicing Yoga. They all underwent complete physical examination and has no disease or medication affecting autonomic nervous system (ANS) in their history. All subjects agreed to participate in the research prior to their inclusion in the study and the consent of ethical committee was obtained and approved the study protocol.
Experimental Procedure and Methods
The experiments were carried out noninvasively as shown in Figure 1 for fifteen PTBI patients Yoga Practicing for at least 12 months (mean age 28.6 years) and fifteen PTBI patients without Yoga Practicing (mean age 30.3 years). The patient was placed on a bed with room temperature of 22C and rested for 10 minutes then he requested in this supine position to raise and lower his leg according to 7 periods of time(frequencies) namely :5s, 10s, 15s, 20s, 25s, 35s and 40s. These periods covered the frequency response of the system for exact identification for exact identification of the system using algorithm by Fakhouri [14]. This algorithm proposed another approach using system identification of the input–output relationship of ant physical or physiological system. This is performed by means of a mathematical model which can be expressed either by a set of differential equations (parameters and static estimation) where the topology of the system is assumed known or by integral equations (non-parametric, weighting function, kernel or functional) which needs little or no prior assumptions about the system. This provides a powerful tool for identification of system whose underlying processes are not well understood
The experiments were carried out noninvasively as shown in Figure 1 for fifteen PTBI patients Yoga Practicing for at least 12 months (mean age 28.6 years) and fifteen PTBI patients without Yoga Practicing (mean age 30.3 years). The patient was placed on a bed with room temperature of 22C and rested for 10 minutes then he requested in this supine position to raise and lower his leg according to 7 periods of time(frequencies) namely :5s, 10s, 15s, 20s, 25s, 35s and 40s. These periods covered the frequency response of the system for exact identification for exact identification of the system using algorithm by Fakhouri [14]. This algorithm proposed another approach using system identification of the input–output relationship of ant physical or physiological system. This is performed by means of a mathematical model which can be expressed either by a set of differential equations (parameters and static estimation) where the topology of the system is assumed known or by integral equations (non-parametric, weighting function, kernel or functional) which needs little or no prior assumptions about the system. This provides a powerful tool for identification of system whose underlying processes are not well understood
So, by using the algorithm [14], it is possible to identify HRV signal-Raising and lowering leg system in terms of the functional Volterra series in which the form of integral equation is fixed and the identification method reduces to the determination of the values within the integral, called kernel. Further details will be found in [14].
The subjects’ signals were recorded for 10 minutes for every period of time mentioned above. These signals include Electrocardiogram (ECG) measured in Lead II sampled at rate of 1000 Hz, HRV (derived from ECG) and stimulus input pulse measured using strain gage mounted on the leg so that electrical pulse produced with the period of time matching to the time of raising and lowering of leg as shown in Figure 1. HRV signals and stimulus input signals were processed through digital filter with bandwidth in the range of 0-1.5 Hz which covered the spectrum of HRV signal stimulated by the periods of lowering and raising a leg and sampled at 3.8 Hz with 1024 points stored for each signal. Figure 2 illustrated the derivation of HRV signal from ECG. The technique used in this study to produce HRV signals based on the hardware described by Cohen., et al. [15] and developed by the author for interfacing to laptop computer. This technique based on hardware device to detect R-R intervals using threshold circuit and interfaced the R-R intervals to software program to reconstruct the heart rate variability signals which is now suitable for sampling and processing as shown in Figure 2. [16]
Results
Heart rate variability signal
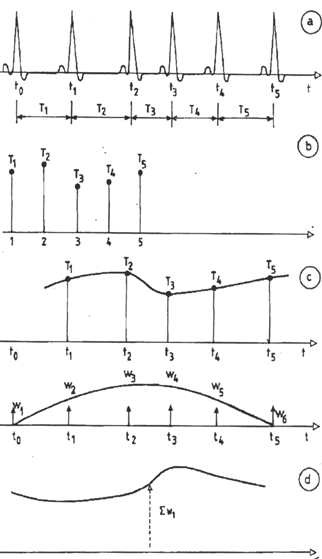
Figure 2: Derivation of heart rate variability (HRV) signal from.
Electrocardiogram (ECG). (a) ECG (b) Detection of R-R interval (c) Construction of HRV signal (d) Smoothed Derivation HRV signal
Electrocardiogram (ECG). (a) ECG (b) Detection of R-R interval (c) Construction of HRV signal (d) Smoothed Derivation HRV signal
Model Order | |||
First Order (NMSE) | Second Order (NMSE) | P value | |
PTBI Non Yoga (15) | 34.50 ± 8.53 | 22.46 ± 5.34 | < 0.05 |
PTBI Yoga Patients (15) | 24.43 ± 11.45 | 16.75 ± 9.76 | 0.001 |
Table 1: Comparison of averaged Normalized Mean Square Errors (NMSE)
of first order and second order kernel prediction of both groups.
Following Fakhouri’s algorithm [14], it is possible to compute the first kernel (impulse response) and second order kernel (mesh
diagram) for both groups. Figures 3 and 4 show the first order kernel for A typical PTBI Yoga practicing patient and A typical PTBI
patient without Non Yoga practicing patient respectively. While Figure 5 illustrates A typical second order kernel of PTBI yoga practicing
and Figure 6 shows A typical second order kernel of TBI patient without Yoga practicing. Table 1 shows comparison of averaged
Normalized Mean Square Errors of HRV variability (NMSE) ± Standard Deviation (in %) of first order and second order rejection as
well as test of significance (p values) for both two groups. Full information about the derivation and statistical analysis of NMSE can
be found in [16]
Discussion
Figure 3 and Figure 4 demonstrate A typical response of the linear part of the system (first order kernel) for both PTBI patient Yoga practicing and PTBI patient without Yoga practicing respectively. This response exhibits the oscillatory and underdamped nature of the system for PTBI practicing Yoga and less oscillatory amplitudes for PTBI patient without practicing Yoga. Also, for mesh diagram as shown in Figures 5 and 6 where the amplitudes of Figures 6 belong to typical PTBI patient without practicing Yoga is greatly reduced compared with Figure 5 of typical PTBI practicing Yoga. This may be attributed to the nature of the system being sensitive to stimulus [11] i.e., the lowering and raising the leg causes variations in heart rate reflected in high response in Yoga practicing PTBI patient as illustrated in figures 3 and 5.
While this process is not happened for PTBI patients without Yoga practicing due to malfunction of autonomic nervous system and well-illustrated in low amplitude in Figures 4 and 6 respectively. The appearance of low amplitudes of second order kernel of figure 6 (mesh diagram) for A typical PTBI patient without Yoga practicing suggests correlation between autonomic function and this diagram which may be used as indicator of the dysfunction of autonomic nervous system in PTBI patients. The significance of Mesh figure (Figure 5) as illustrated for Yoga practicing PTBI is to give insight the dynamics of the effect of Yoga in autonomic nervous system in this group with high amplitude with respect to the other group without Yoga practicing as illustrated Figure 6 Table 1 summarizes the average NMSE of the model prediction for 15 PTBI patient practicing Yoga and 15 PTBI patients without Yoga practicing. It is apparent that NMSE of the first order model is about 8-12 % higher than NMSE of the second order model for both groups. This indicated the significance of second order model of the system in describing the nonlinearity and complexity of relationship between stimulus and HRV. Referring to Table 1, The NMSE for first order and second order for PTBI patients without Yoga practicing is greater than PTBI patients Yoga practicing (p < 0.05 and 0.001 respectively). These results may give model quantitative indices to assess the autonomic nervous system in health and disease.
This study demonstrates the significance of using the Kernal method and entrainment technique in assessing the function of autonomic nervous system for Yoga practicing PTBI patients compared to non practicing Yoga PTBI patients. However, further study is required to investigate more Yoga Practicing PTBI patients as well as using other quantitative methods to identify the prognosis of autonomic function with duration of Yoga practicing.
Acknowledgement
The author would like to appreciate the cooperation and collaboration of Department of neurology at Johns Hopkins Hospital, Baltimore, MD, USA for facilitating the measurement of physiological signals. Also, I appreciate Mr. Karim Ahmed for his help in statistical analysis, Ms Fatma Ibrahim for Yoga instruction and Ms Voris for her guidance instructions for PTBI patients. The processing and analysis of the signals as well as developing the algorithms were carried out at Tennessee Tech University, Cookeville, TN 38501, USA.
The author would like to appreciate the cooperation and collaboration of Department of neurology at Johns Hopkins Hospital, Baltimore, MD, USA for facilitating the measurement of physiological signals. Also, I appreciate Mr. Karim Ahmed for his help in statistical analysis, Ms Fatma Ibrahim for Yoga instruction and Ms Voris for her guidance instructions for PTBI patients. The processing and analysis of the signals as well as developing the algorithms were carried out at Tennessee Tech University, Cookeville, TN 38501, USA.
References
- JG Van Dijk., et al. “Autonomic nervous system dysfunction in Parkinson’s disease: relation with age, medication, duration and severity”. Journal of Neurology, Neurosurgery, and Psychiatry 56.10 (1993): 1090-1095.
- Ryan ML., et al. “Heart rate variability is an independent predictor of morbidity and mortality in hemodynamically stable trauma patients”. The Journal of Trauma 70.6 (2011): 1371-1380.
- Muralikrishnan K., et al. “Measurement of the effect of Isha Yoga on cardiac autonomic nervous system using short-term heart rate variability”. Journal of Ayurveda and Integrative Medicine 3.2 (2012): 91-96.
- Hinson HE., et al. “Early Fever as a Predictor of Paroxysmal Sympathetic Hyperactivity in Traumatic Brain Injury”. The Journal of Head Trauma Rehabilitation (2017).
- Laxe S., et al. “How does dysautonomia influence the outcome of traumatic brain injured patients admitted in a neurorehabilitation unit?” Brain Injury27.12 (2013): 1383-1387.
- Fernandez-Ortega JF., et al. “Paroxysmal sympathetic hyperactivity after traumatic brain injury: clinical and prognostic implications”. Journal of Neurotrauma 29.7 (2012): 1364-1370.
- Ryan ML., et al. “Heart rate variability is an independent predictor of morbidity and mortality in hemodynamically stable trauma patients”. Journal of Trauma 70.6 (2011): 1371-1380.
- Mourya M., et al. “Effect of slow- and fast-breathing exercises on autonomic functions in patients with essential hypertension”. Journal of Alternative and Complementary Medicine 15.7 (2009): 711-717.
- Rajani SN., et al. “Role Of Yoga On Cardic Autonomic Function Tests And Cognition In Type 2 Diabetes”. International Journal of Research in Ayurveda and Pharmacy 6.6 (2015): 764-766.
- C Singer WJ., et al. “Autonomic dysfunction in men with Parkinson’s disease”. European Neurology 32.3 (1992): 134-140.
- A Kamal. “Assessment of autonomic function using complex demodulation and posture entrainment techniques: an application to normal subjects and diabetic patients”. Frontiers of Medical & Biological Engineering Journal 7.1 (1996): 1-10.
- JT Korpelainen., et al. “Dynamic behavior of heart rate in ischemic stroke”. Stroke 30.5 (1999): 1008-1013.
- M Pagani., et al. “Relationship between spectral components of cardiovascular variabilities and direct measures of sympathetic activity in humans”. Circulation 95.6 (1997): 1441-1448.
- SY Fakhouri. “Identification of Volterra kernels of nonlinear discrete system”. IEE Proceedings - Control Theory and Applications 127 (1980): 296-304.
- AJ Cohen O. “Measurement of heart rate variability, part 2- hard digital device for assessment of heart rate variability”. Medical Biological Engineering Company 15 (1977): 423-430.
- K Shafqat. et al. “Empirical mode decomposition (EMD) analysis of HRV data from locally anesthetized patients”. Annual International Conference of the IEEE Engineering in Medicine and Biology Society (2009): 2244-2247.
Citation:
Ahmed K Kamal. “Autonomic Function Assessment in Post Traumatic Brain Injury patients with Yoga Practicing Using Kernel
Method and Entrainment Techniques”. Current Opinions in Neurological Science 1.1 (2017): 74-80.
Copyright: © 2017 Ahmed K Kamal. This is an open-access article distributed under the terms of the Creative Commons Attribution License, which permits unrestricted use, distribution, and reproduction in any medium, provided the original author and source are credited.