Research Article
Volume 1 Issue 6 - 2017
Evaluation and Optimisation of in Silicon Designed B-Secretase Modulators for the Treatment of Alzheimer’s Disease
Department of Pharmacy, Faculty of Medicine and Surgery, University of Malta
*Corresponding Author: Luke Borg, Department of Pharmacy, Faculty of Medicine and Surgery, University of Malta.
Received: November 11, 2017; Published: November 28, 2017
Abstract
Alzheimer's disease affects cognitive function through formation of ß- secretase mediated extracellular cerebral protein plaques and intracellular neurofibrillary tangles, thus its antagonism could mitigate disease progression. This project aims to identify newly obtained and optimized molecules which decrease the formation of ß -amyloid plaques through inhibition of the ß- secretase enzyme. Protein databank (PDB) depositions describing the bound coordinates of 6 lead structures complexed with ß- secretase were identified (PDB ID- 2VKM, 4B05, 4IVS, 3U6A, 3IGB, 2Q11) as leads for in silico ligand based and de novo design of novel antagonist molecules. For the first part of this study, ligands extracted from the protein were used as templates for screening ViCi Hamburg’s database.
Protomols were generated for each of the ligands using the Surflex Dock suite in SYBYL-X. The molecules received through ViCi were then used as ligand sources. For the second part of the study the ligand binding affinity (LBA) of each small molecule for its cognate receptor was calculated in X-Score for baseline affinity establishment. 2D topology maps highlighting the important interactions between ligand and receptor were generated using Poseview, and noncritical moieties were computationally removed in the process of creating seed structures (n = 3,2,3,2,2,2 respectively) on to which novel moieties were computationally introduced using the GROW module of LigBuilder. Protomol and Keysite volumes were then compared using UCSF Chimera. 1636 novel structures were generated with 253 structures being Lipinski Rule compliant. The highest ranking molecules from each pharmacophoric family were identified for optimization and in vitro validation.
Keywords: Beta- Secretase; BACE1; Alzheimer’s disease; De novo drug design; In silico ligand- based drug design
Introduction
Alzheimer’s disease (AD) is the most common form of dementia, where the brain is physically damaged, affecting an individual’s daily activities, which relate to memory, thinking and behavior. As defined by the Alzheimer’s Association, AD is a degenerative disease; which means that gradually, more parts of the brain become damaged. As this happens, the symptoms become more severe [1].
AD was first described by the German neurologist Alois Alzheimer, as a physical disease which affects the brain. As the disease progresses, extracellular protein plaques (amyloid-beta proteins) and intracellular neurofibrillary tangles (microtubule associated tao proteins) develop in different parts of the brain, leading to the death of brain cells. People with Alzheimer's also have a shortage of some important chemicals, which are important for the transmission of messages within the brain. Persons with AD may experience various symptoms. The symptoms of AD and allied regression do not manifest uniformly amongst patients, however there are recognized general stages, based on a system developed by Reisberg, which divides the progress of the disease symptoms into 7 stages [2].
There is still no recognized curative treatment or medication for curing AD, however the symptoms including memory loss, anxiety, behavioral changes; may be controlled by some drugs. The most frequently used drugs are cholinesterase inhibitors. As the name implies, they inhibit the breakdown of acetylcholine, a neurotransmitter important for electric transmission in neurons, thus reducing memory degeneration.
“It is generally recognized that an excess level of amyloid- Beta (Aβ) in the brain over a long time period is a leading factor in the pathogenesis of Alzheimer’s Disease (AD)” (Selkoe and Schenk, 2008) [3]. β-Secretase, also known as BACE1, is a transmembrane aspartic protease that makes specific cleavages during the maturation of some protein chains. These types of proteins are known as trimming proteins. The enzyme is normally found in the endoplasmic reticulum and in Golgi Bodies, where it trims proteins important to neuronal function.
These include the protein neuregulin, which helps in the control of the formation of myelin sheaths around nerve axons, and voltage-gated sodium channels, which are important for the transmission of nerve signals. The BACE1 is structurally similar to the digestive protease pepsin. They both have a deep active site cleft which grips the protein chains, and a pair of aspartate amino acids which make the cut. The difference between the two is that BACE1 has a long tail that attaches it to the membrane surface, so that the enzyme cannot float freely around the cell, causing cleavage of other important proteins which should not be cleaved [4].
BACE1, however, also makes a specific cut in the amyloid-beta precursor protein (APP), breaking the protein chain and releasing a large portion of it, which explains why it is also known as BACE1- β-site amyloid precursor protein cleaving enzyme 1. A second protease known as γ-secretase then cleaves the other side of the protein, and a small, dangerous peptide, known as the amyloid- β, is subsequently formed. In small amounts, this peptide is important for synaptic function. However, if BACE1 is overactive, this peptide builds up and can combine into tangled fibers. If these fibers form in nerve cells, they can block nerve transmission which leads to AD. This is the reason why BACE1 is currently recognized as one of the major targets for the management of AD.
The BACE1 active site is located within the extracellular domain of the protein. Its active site is made up of two aspartate residues- Asp32 and Asp228 hence its classification as an aspartic protease (Figure 1). This is due to its nature as an aspartyl protease. The R groups of both aspartates coordinate a single water molecule between the two, allowing for a nucleophilic attack to occur at the carbonyl groups [4].
This project consequently aims to identify newly obtained and optimized molecules, which inhibit, or decrease the formation of the β- amyloid plaques through inhibition of the BACE1, with potential for clinical use in the therapeutic management of AD.
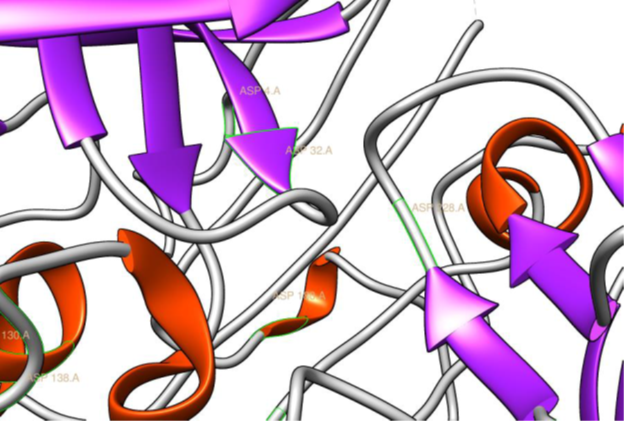
Figure 1: The two aspartyl residues Asp32 and Asp228, present in the
active site of BACE1, based on the co-ordinates of PDB ID 2VKM (Ghosh.,
et al. 2008), rendered in Chimera (Pettersen EF., et al. 2004) (21).
Materials and Methods
Six X-ray crystallographic depositions describing the bound co-ordinates of six ligands with BACE1 were selected from the Protein Data Bank (PDB) [5]. These were PDB ID 2VKM describing the holo- BACE1 bound to its cognate ligand GRL-8234 (Ghosh., et al. 2008) [6], PDB ID 4B05, describing the bound co- ordinates of the holo- BACE1 to the experimental drug AZD3839 (Jeppsson., et al. 2012) [7], and PDB ID 4IVS, describing the holo- BACE1 to the indole acylguanidine inhibitor (Zou., et al. 2013) [8], PDB ID 3U6A describing the holo- BACE1 bound to an aminopiperazinone ligand (Tresadern., et al. 2011) [9], PDB ID 3IGB describing the holo- BACE1 bound to an aminoimidazole ligand (Malamas., et al. 2009) [10], and PDB ID 2Q11 describing the holo- BACE1 bound to an aminoquinazoline (Baxter., et al. 2007) [11]. Each deposition was visualized and edited in SYBYL®- X 1.2 [12] molecular modeling software, prior to Ligand Binding Affinity (LBA) estimation. Water molecules at a distance ≥ 5 Å from the BACE1_LBP were deleted. At the end of the process a file saved in .mol2 format containing the bound co-ordinates of the extracted small molecules and another file saved in .pdb format containing the apo receptor, without the deleted water molecules were generated.
Part 1 – in silico ligand- based drug design
The online freeware, ViCi (13) was used for screening the extracted ligands, GRL_8234.mol2, AZD3839.mol2, ACYLGUAN.mol2, 18P.mol2, CMPND3.mol2, Structure1.mol2 from PDB crystallographic depositions 2VKM, 4B05, 4IVS, 3U6A, 3IGB, and 2Q11 (6-11) respectively.The six .mol2 files were converted to .pdb format using SYBYL®- X 1.2 (12). They were subsequently used as templates for the ViCi [13] screening process.
The online freeware, ViCi (13) was used for screening the extracted ligands, GRL_8234.mol2, AZD3839.mol2, ACYLGUAN.mol2, 18P.mol2, CMPND3.mol2, Structure1.mol2 from PDB crystallographic depositions 2VKM, 4B05, 4IVS, 3U6A, 3IGB, and 2Q11 (6-11) respectively.The six .mol2 files were converted to .pdb format using SYBYL®- X 1.2 (12). They were subsequently used as templates for the ViCi [13] screening process.
A protomol was generated using SYBYL®- X 1.2 (12) for each of the six ligands. The six original PDB files 2VKM, 4B05, 4IVS, 3U6A, 3IGB and 2Q11 (6-11) were retrieved from the RCSB server through SYBYL®- X 1.2 (12). The process described below was therefore performed for each of the six PDB depositions.
The ligand was extracted from the protein backbone, while the water molecules together with any other ions deemed inessential for ligand stability were removed from the protein. To generate the protomol, the area occupied by the ligand (the active site) was used as placement for the Surflex-Dock process.
The molecules that were identified from the molecular database ViCi (13) during the virtual screening process in .pdb format, were converted into a single .sdf format file using Mona (Hilbig and Rarey, 2015) [14]. 7 Lipinski Rule compliant decoy molecules were chosen arbitrarily and added to the others in the same .sdf file. Ligand filtering was performed in Mona (14), in order to ensure that only Lipinski Rule compliant ligands would be considered further. These were saved as a single .sdf file. This process was performed for each of the six ligands, resulting in five .sdf files, namely containing Lipinski Rule compliant molecules with potential to act as ligands in the protomol owing to the fact that one of the six template molecules produced a cohort of molecular structures that were all Lipinski Rule non-compliant.
Each of the .sdf files prepared were chosen separately as Ligand Sources, and the Surflex Dock process was run for each PDB crystallographic deposition, except 2VKM (6), which resulted in no Lipinski Rule compliant molecules.
Part 2 - de novo drug design
In this step the extracted ligand molecules in .mol2 format and their respective protein binding pocket .pdb files, from part 1, were transferred to X-Score® v1.3 (Wang., et al. 1998) [15], which runs on a Linux Platform. The X-Score® v1.3 (15) algorithm was used to quantify (pKd) the LBA of each ligand for its cognate receptor. This process was done to establish a baseline affinity against which the LBAs of other seeds would then be compared.
In this step the extracted ligand molecules in .mol2 format and their respective protein binding pocket .pdb files, from part 1, were transferred to X-Score® v1.3 (Wang., et al. 1998) [15], which runs on a Linux Platform. The X-Score® v1.3 (15) algorithm was used to quantify (pKd) the LBA of each ligand for its cognate receptor. This process was done to establish a baseline affinity against which the LBAs of other seeds would then be compared.
A thorough study of the structure activity relationship for PDB depositions 2VKM, 4B05, 4IVS, 3U6A, 3IGB, and 2Q11 (6-11) was carried out. Important moieties listed in each of the six ligands’ published articles were retained. Secondly PoseView v1.1.2 [16] was used in order to facilitate SAR understanding by generating 2D topology maps. All bonds and interactions marked as critical to binding by PoseView v1.1.2 (16) were taken into consideration when creating seeds so as to try not disrupt the moieties essential to binding. Thus, moieties which were deemed not important for binding, were selected as sites for molecular growth, while moieties deemed important to ligand stability and affinity were retained as much as possible. Seed structures were then created for each of the six resident ligands, based on the acquired SAR.
de novo design was carried out using LigBuilder® v1.2 (Wang., et al. 2000) [17] suite, which is divided into 3 modules namely POCKET, GROW and PROCESS. The POCKET module, identifies pharmacophoric structural features and key interaction sites that a hypothetical ligand for a given Ligand Binding Pocket (LBP) could occupy. In this study, the PDB version of the apo- BACE1 (Patel., et al. 2004) [18] was investigated and the bioactive conformation of the six ligands selected (saved in .mol2 format) served as a probe in order to elucidate the 3-D volume of BACE1_LBP and to generate a basic pharmacophoric structure for ligands of this bindingpocket.In this study, each of the six ligands extracted from the six PDB files in .mol2 format were used to probe the cognate receptor in PDB file format according to the POCKET algorithm. The resulting data was then used by the GROW module of LigBuilder® v1.2 (17) through which de novo design was actually performed. The next process of the study utilized the GROW module of LigBuilder® v1.2 (17). This module has the ability to construct ligand molecules for a target protein in this case BACE1, by applying the inbuilt growing strategy algorithm. This growing strategy is contingent on the provision of appropriate seed structures. In this step of the study the seeds were allowed to grow alternative side chains. Each of the designed seed structures saved in mol2 format were transferred to LigBuilder® v1.2 (17) for use in the GROW phase of the de novo design study. Each of the 14 seeds were made up of only one fragment, therefore the GROW strategy was used to guide unidirectional molecular growth.
An assessment to predict the drug-likeness for the de novo ligands was carried out based on the parameters of Lipinski’s Rule of 5 (Lipinski., et al. 2001) [19] by transferring each individual de novo ligand to Discovery Studio v4.0, which determined the compliance of such de novo ligands to Lipinski’s Rule of 5 based on the following parameters:
- - Number of Hydrogen bond donors ≤ 5
- - Number of Hydrogen bond acceptors ≤ 10
The compliance of the seeds to the other two parameters, namely
- - Molecular weight < 500
- - Octanol – water partition coefficient ≤ 5
Was determined by choosing the seeds from each of the index files obtained from LigBuilder® v1.2 (17) after performing the GROW strategy as mentioned in the previous section.
Comparison
The Protomol and Keysite volumes were calculated and analyzed using SYBYL®- X 1.2 (12) by loading each protomol and keysite into SYBYL®- X 1.2 (12) consecutively and running the ‘Multiple Volume’ process for each one.
The Protomol and Keysite volumes were calculated and analyzed using SYBYL®- X 1.2 (12) by loading each protomol and keysite into SYBYL®- X 1.2 (12) consecutively and running the ‘Multiple Volume’ process for each one.
Results and Discussion
In silico ligand- based drug design results
A protomol was generated for each of the six chosen PDB crystallographic depositions of the BACE1 using SYBYL®- X 1.2 (12) Surflex Dock process- 2VKM, 4B05, 4IVS, 3U6A, 3IGB, and 2Q11 (6-11). The number of Lipinski Rule compliant molecules for each PDB file received by ViCi (13) are shown in Table 1. The Surflex Dock Process was performed and resulted in a total of 2736 Lipinski Rule compliant molecules. The top 6 ligands with the highest Total Score are shown in Table 2.
A protomol was generated for each of the six chosen PDB crystallographic depositions of the BACE1 using SYBYL®- X 1.2 (12) Surflex Dock process- 2VKM, 4B05, 4IVS, 3U6A, 3IGB, and 2Q11 (6-11). The number of Lipinski Rule compliant molecules for each PDB file received by ViCi (13) are shown in Table 1. The Surflex Dock Process was performed and resulted in a total of 2736 Lipinski Rule compliant molecules. The top 6 ligands with the highest Total Score are shown in Table 2.
PDB ID | 2VKM | 4B05 | 4IVS | 3U6A | 3IGB | 2Q11 |
Lipinski Rule Compliant ViCi Ligands | 0 | 357 | 658 | 626 | 487 | 608 |
Total Lipinski Rule Compliant Ligands including 7 decoy molecules | 0 | 364 | 665 | 633 | 494 | 615 |
Table 1: Lipinski Rule Compliant Molecules for each set of ViCi Results.
Table 2: Top 6 ligand molecules with the highest Total Score.
De novo drug design results
Table 3 depicts the LBA (pKd) of the six ligands GRL-8234 (PDB ID 2VKM) (6), AZD3839 (PDB ID 4B05) (7), the indole acylguanidine (PDB ID 4IVS) (8), the aminopiperazinone (PDB ID 3U6A) (9), the aminoimidazole (PDB ID 3IGB) (10), and the aminoquinazoline (PDB ID 2Q11) (11). These baseline affinities were obtained using X-Score® v1.3 (15) by loading apo- BACE1 (18) in .pdb format and docking the ligands in .mol2 format in the BACE1_LBP.
Table 3 depicts the LBA (pKd) of the six ligands GRL-8234 (PDB ID 2VKM) (6), AZD3839 (PDB ID 4B05) (7), the indole acylguanidine (PDB ID 4IVS) (8), the aminopiperazinone (PDB ID 3U6A) (9), the aminoimidazole (PDB ID 3IGB) (10), and the aminoquinazoline (PDB ID 2Q11) (11). These baseline affinities were obtained using X-Score® v1.3 (15) by loading apo- BACE1 (18) in .pdb format and docking the ligands in .mol2 format in the BACE1_LBP.
Ligand | in silico LBA (pKd) |
GRL-8234 (PDB ID: 2VKM) | 8.86 |
AZD3839 (PDB ID: 4B05) | 7.07 |
Indole Acylguanidine (PDB ID: 4IVS) | 6.16 |
Aminopiperazinone (PDB ID: 3U6A) | 6.38 |
Aminoimidazole (PDB ID: 3IGB) | 5.98 |
Aminoquinazoline (PDB ID: 2Q11) | 6.76 |
Table 3: Predicted in silico LBA (pKd) of the six template ligands for BACE1.
Ligand Generation-
Seed structures were created for each of the six ligands as follows-
- 3 seed structures created from PDB ID 2VKM (6) ligand GRL-8234
- 2 seed structures created from PDB ID 4B05 (7) ligand AZD3839
- 3 seed structures created from PDB ID 4IVS (8) indole acylguanidine ligand
- 2 seed structures created from PDB ID 3U6A (9) aminopiperazinone ligand
- 2 seed structures created from PDB ID 3IGB (10) aminoimidazole ligand
- 2 seed structures created from PDB ID 2Q11 (11) aminoquinazoline ligand
Table 4 shows the number of molecules and their respective families generated from the respective seed structure.
Seed structures were created for each of the six ligands as follows-
- 3 seed structures created from PDB ID 2VKM (6) ligand GRL-8234
- 2 seed structures created from PDB ID 4B05 (7) ligand AZD3839
- 3 seed structures created from PDB ID 4IVS (8) indole acylguanidine ligand
- 2 seed structures created from PDB ID 3U6A (9) aminopiperazinone ligand
- 2 seed structures created from PDB ID 3IGB (10) aminoimidazole ligand
- 2 seed structures created from PDB ID 2Q11 (11) aminoquinazoline ligand
Table 4 shows the number of molecules and their respective families generated from the respective seed structure.
PDB ID | Seed Number | Number of Famillies | Number of Molecules | Highest pKd |
2VKM | 1 | 1 | 1 | 5.28 |
2 | 1 | 2 | 5.95 | |
3 | 5 | 8 | 5.98 | |
4B05 | 1 | 1 | 1 | 5.18 |
2 | 6 | 14 | 5.97 | |
4IVS | 1 | 18 | 200 | 9.76 |
2 | 23 | 200 | 9.88 | |
3 | 4 | 10 | 6.35 | |
3U6A | 1 | 12 | 200 | 8.87 |
2 | 21 | 200 | 6.72 | |
3IGB | 1 | 8 | 200 | 9.95 |
2 | 9 | 200 | 8.35 | |
2Q11 | 1 | 14 | 200 | 9.99 |
2 | 8 | 200 | 10.00 |
Table 4: Number of novel structures generated from each seed.
Top Lipinski Rule compliant de novo ligands with a higher LBA than the template ligand, for each seed structure are represented in Table 5.
Table 5: Table showing the top Lipinski Rule compliant ligands with a higher LBA than the template ligand, for each seed structure.
Protomol volumes and Keysite volumes calculated in SYBYL®- X 1.2 (12) are shown in Table 6. The volumes were calculated using SYBYL®- X 1.2 (12).
PDB Deposition | Key site Volume (Å3) | Protomol Volume (Å3) |
2VKM | 905 | 1538 |
4B05 | 683 | 897 |
4IVS | 858 | 1244 |
3U6A | 179 | 914 |
3IGB | 257 | 572 |
2Q11 | 385 | 1013 |
Table 6: Comparison of key site and protomol volumes (ų).
Discussion
De novo design may be defined as the design of lead compounds by adding moieties to a molecule, within a model of the target receptor or active site, the structure of which is already known. This approach thus attempted to identify novel inhibitors for the BACE1 active site that were also Lipinski Rule compliant. Seed structures were created for each PDB crystallographic deposition selected with the aim to grow ligands with even higher affinities than the original lead compounds.
The three seed structures created from GRL-8234 (6) resulted in a total of 7 families. Since none of the molecules were Lipinski Rule compliant and none had a higher LBA than the original ligand they were deemed not important for further optimisation. For seed 1 LigBuilder® v1.2 (17) added a methyl (-CH3) moiety to the growing site, causing Result 1 to have a lower affinity than the original GRL-8234 ligand (6) so adding this moiety was deemed to decrease the LBA. For seed 2, Result_001 had a lower logP and the higher LBA from the two resulting molecules, making it the better result generated from this seed.
The addition of 2 methyl moieties in each molecule resulted in two different binding affinities so this was deemed to be caused by the different spatial placement of the added moieties. Seed 3 generated 5 families with a total of 8 molecules, with Result 1 from Family 1 being the best and Result 7 from Family 4 being the worst in LBA, hence the pharmacophore for family 1 was deemed to contribute to a greater LBA than that of Family 4.
The two seed structures created from AZD3839 (7) resulted in a total of 7 families containing 15 molecules in all. The two Lipinski Rule compliant molecules obtained, both from seed 2, one from family 1, and the other from family 3 unfortunately had a lower LBA than the original binding affinity for ligand AZD3839 so they were also deemed not satisfactory for further optimisation.
The moiety grown in LigBuilder® v1.2 (17) for seed 1, Result 1, contributed to a lower LBA. For seed 2, the two Lipinski Rule compliant molecules generated, had a logP between 3 and 5, which is within parameters for Lipinski Rule of Five compliancy, however, with a LBA of 5.61 (Result 1) and 5.38 (Result 8) they both had lower LBA than the original ligand AZD3839 (7).
The three seed structures created from indole acylguanidine (8) resulted in a total of 410 molecules generated using LigBuilder® v1.2 (17). The two Lipinski Rule compliant molecules obtained were from seed 1, family 2, and the other from seed 3, family 9. The LBA of the ligand from seed 1, family 2 (Result 72) was both Lipinski Rule compliant and also had a higher LBA (pKd = 7.72) than the original molecule (pKd = 6.16) so the moiety grown by LigBuilder® v1.2 (17) was seen to contribute to an increased LBA and thus deemed to be a valid candidate for further optimisation. LogP was 4.93, which is within the parameters set by Lipinski’ s Rule of Five [19], so all calculated values were within the parameters for a CNS acting drug (Ghose., et al. 2012) [20] with high affinity for BACE1. Result 1 from Family 1 had the highest LBA of 9.76 however was not Lipinski Rule compliant, while Result 46 had the lowest LBA of 7.71 from Family 1 and was also not Lipinski Rule compliant.
200 novel structures were generated from each seed of the aminopiperazinone, aminoimidazole and aminoquinazoline class. The first aminopiperazinone seed yielded molecules from 6 different families. The second aminopiperazinone seed yielded molecules from 12 different families. The families differed from one another by having a different pharmacophoric scaffold. 27.5% of the novel molecules generated from the aminopiperazinone seeds were found to be Lipinski rule compliant (19). Seed 1 from the aminoimidazole class yielded novel structures from 6 different families. The second aminoimidazole seed generated molecules from 9 different families. Compared to the structures generated from the other 2 classes considered, the novel molecules yielded from the aminoimidazole seeds showed a sharp increase in Lipinski rule compliance, with 88% of the total generated structures being compliant.
The first seed from the aminoquinazoline class yielded molecules from 6 different families whilst the second aminoquinazoline seed yielded molecules from 4 different families. Only 7.5% of the total novel aminoquinzoline-derived structures were found to be Lipinski compliant.
The in silico ligand- based drug design method resulted in discovering new molecules, which were previously not studied in the BACE1 active site. A number of Lipinski Rule compliant molecules gave a Total Score of 6 or higher. This part of the study may also be considered to take a more innovative approach than the de novo process due to the larger surface area and volume explored through the creation of the protomol. As can be seen in the results section, the protomols created by SYBYL®- X 1.2 (12) all had a larger surface area and volume than the LBP map created by LigBuilder® v1.2 (17). This allowed for more robust exploration of the active site, thus providing more possible interaction sites than the LBP map generated by LigBuilder® v1.2 (17).
Analysis of the top six structures shed light on important properties. Molecule Asinex_ASN_05381986, which exhibited the highest Total Score interacts with the active site through many different favorable interactions. The only unsatisfied moieties are the nitrogen atoms in the tetrazolidine moiety.
0058_Chemti_LT-1204_X_0039, which exhibited a Total Score of 7.63 and also interacts with the BACE1 active site through many favorable interactions. The unsatisfied moieties are two oxygen atoms. 0215_Amri_ALB-H00728600 exhibited a Total Score of 7.61 and also interacts with the BACE1 active site through many favorable interactions. The unsatisfied moieties are two hydrogen atoms and an oxygen atom.
Chembridge_11204462, which exhibited a Total Score of 7.53 also interacts with the BACE1 active site through many favorable interactions. The unsatisfied moieties are mainly nitrogen and oxygen atoms. 0716_Chemti_NPP1152, which exhibited a Total Score of 7.45 interacts with the BACE1 active site through many favorable interactions. The unsatisfied moieties are mainly nitrogen, oxygen and hydrogen atoms. Amri_ALB-H00452300 exhibited a Total Score of 7.39 and also interacts with the BACE1 active site through many favorable interactions. The unsatisfied moieties are three oxygen atoms.
Limitations
As with any in silico drug design study, the project was conducted using a static, rigid LBP, which is different from in vivo modeling and may thus yield different results. This was a limitation of the freeware used and of SYBYL®- X 1.2 (12) which could not be overcome.
As with any in silico drug design study, the project was conducted using a static, rigid LBP, which is different from in vivo modeling and may thus yield different results. This was a limitation of the freeware used and of SYBYL®- X 1.2 (12) which could not be overcome.
Conclusion
In further studies the primary recommendation would be to optimize further the top ranking molecules from both cohorts, and also to then allow both enzyme and ligand to adopt complementary conformations to each other upon binding. Although a time consuming process, invaluable information can be achieved. The yielded results would then pave the way for further molecular dynamic evaluation and in vitro assay together with further necessary structural optimisation.
References
- Latest Alzheimer's Facts and Figures [Internet]. Latest Facts & Figures Report | Alzheimer's Association. 2013 [cited 18 September 2016].
- Stages of Alzheimer's & Symptoms | Alzheimer's Association [Internet]. Alz.org. 2016 [cited 18 September 2016].
- Selkoe DJ. “Soluble oligomers of the amyloid β-protein impair synaptic plasticity and behaviour”. Behavioural Brain Research 192.1 (2008): 106-113.
- Vassar R., et al. “The β-Secretase Enzyme BACE in Health and Alzheimer's disease: Regulation, Cell Biology, Function, and Therapeutic Potential”. Journal of Neuroscience 29.41 (2009): 12787-12794.
- Berman H., et al. “The worldwide Protein Data Bank (wwPDB): ensuring a single, uniform archive of PDB data”. Nucleic Acids Research 35 (2007): 301-303.
- Ghosh AK., et al. “Beta-secretase as a therapeutic target for Alzheimer's disease”. Neurotherapeutics 5.3 (2008): 399-408.
- Jeppsson F., et al. “Discovery of AZD3839, a Potent and Selective BACE1 Inhibitor Clinical Candidate for the Treatment of Alzheimer Disease”. The Journal of Biological Chemistry 287.49 (2012): 41245-41257.
- Zou Y., et al. “Virtual screening and structure-based discovery of indole acylguanidines as potent β-secretase (BACE1) inhibitors”. Molecules 18.5 (2013): 5706-5722.
- Tresadern G., et al. “Rational design and synthesis of aminopiperazinones as β-secretase (BACE) inhibitors”. Bioorganic & Medicinal Chemistry Letters 21.24 (2011): 7255-7260.
- Malamas MS., et al. “Aminoimidazoles as Potent and Selective Human β-Secretase (BACE1) Inhibitors”. Journal of Medicinal Chemistry 52.20 (2009): 6314-6323.
- Baxter EW., et al. “2-Amino-3, 4 dihydroquinazolines as Inhibitors of BACE-1 (â-Site APP Cleaving Enzyme): Use of Structure Based Design to Convert a Micromolar Hit into a Nanomolar Lead”. Journal of Medicinal Chemistry 50.18 (2007): 4261-4264.
- SYBYL-X 1.2 [computer program], Tripos International, 1699 South Hanley Rd., St. Louis, Missouri, 63144, USA;2010.
- Lamzin V. ViCi - in-silico ligand-based drug design [Internet]. Embl-hamburg.de. 2016 [cited 18 September 2016].
- Hilbig M and Rarey M. “MONA 2: A Light Cheminformatics Platform for Interactive Compound Library Processing”. Journal of Chemical Information and Modeling 55.10 (2015): 2071-2078.
- Wang R., et al. “Further Development and Validation of Empirical Scoring Functions for Structure-Based Binding Affinity Prediction”. Journal of Computer-Aided Molecular Design 16.1 (2002): 11-26.
- Stierand K., et al. “Molecular Complexes at a Glance: Automated Generation of two-dimensional Complex Diagrams”. Bioinformatics 22.14 (2006): 1710-1716.
- Wang R., et al. “LigBuilder: A Multi-Purpose Program for Structure-Based Drug Design”. Molecular modeling annual 6.7.8 (2000): 498-516.
- Patel S., et al. “Apo and Inhibitor Complex Structures of BACE (β-secretase)”. Journal of Molecular Biology 343.2 (2004): 407-416.
- Lipinski CA., et al. “Experimental and computational approaches to estimate solubility and permeability in drug discovery and development settings”. Advanced Drug Delivery Reviews 146.1.3 (2001): 3-26.
- Ghose AK., et al. “Knowledge-Based, Central Nervous System (CNS) Lead Selection and Lead Optimization for CNS Drug Discovery”. ACS Chemical Neuroscience 3.1 (2012): 50-68.
- Pettersen EF., et al. “UCSF Chimera--A visualization system for exploratory research and analysis”. Journal of Computational Chemistry 25.13 (2004): 1605-1612.
Citation:
Luke Borg., et al. “Evaluation and Optimisation of in Silicon Designed B-Secretase Modulators for the Treatment of Alzheimer’s
Disease”. Chronicles of Pharmaceutical Science 1.6 (2017): 320-331.
Copyright: © 2017 Luke Borg., et al. This is an open-access article distributed under the terms of the Creative Commons Attribution License, which permits unrestricted use, distribution, and reproduction in any medium, provided the original author and source are credited.